Basic Image Operations¶
[1]:
# Useful libraries
import numpy as np
import cv2
import os
import sys
# Carnot Research Vision Library
from cr import vision
%matplotlib inline
# Images supplied with this library
MODULE_DIR = os.getcwd()
PACKAGE_DIR = os.path.dirname(MODULE_DIR)
DATA_DIR = os.path.join(PACKAGE_DIR, 'data')
IMAGES_DIR = os.path.join(DATA_DIR, 'images')
Creating Simple Images¶
A gray scale blank image
[2]:
black = vision.blank_image(300, 300)
[3]:
vision.imshow(black)
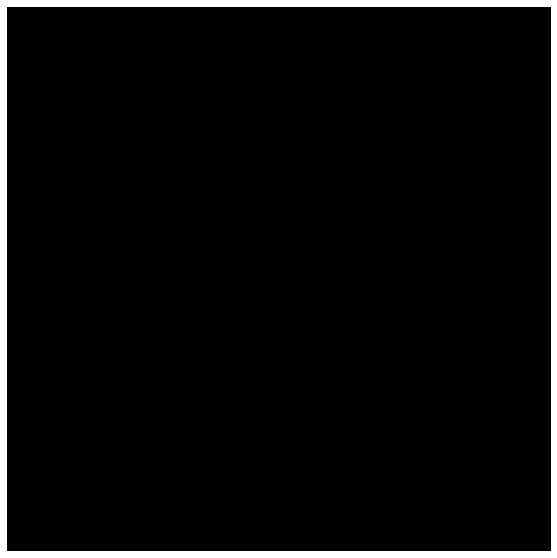
Image Cleanup via Adaptive Thresholding¶
Let’s load an image of a page of text scanned in low light conditions.
[4]:
image_path = os.path.join(IMAGES_DIR, 'bookpage_dark_scan.jpg')
image = cv2.imread(image_path)
vision.imshow(image)
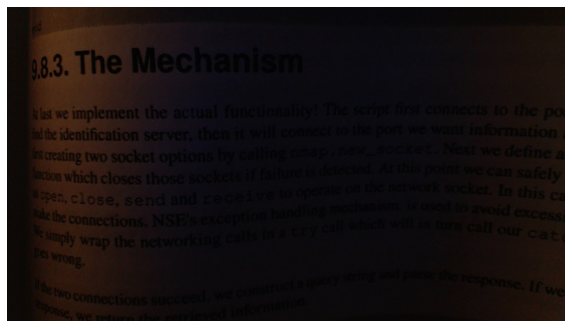
Let’s convert the image to gray scale (note that OpenCV loads images in BGR format)
[5]:
gray_image = vision.bgr_to_gray(image)
Let’s apply an adaptive thresholding algorithm to cleanup the image
[6]:
thresholded_image = vision.adaptive_threshold_gaussian(
gray_image, block_size=115, constant=1)
vision.imshow(thresholded_image)
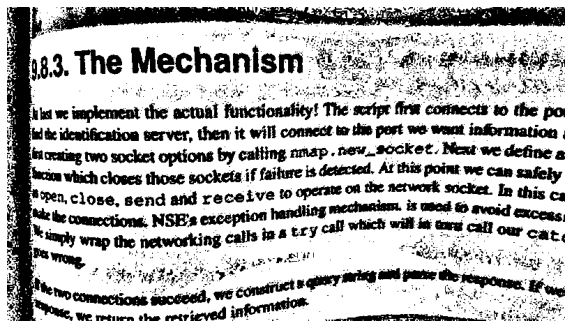